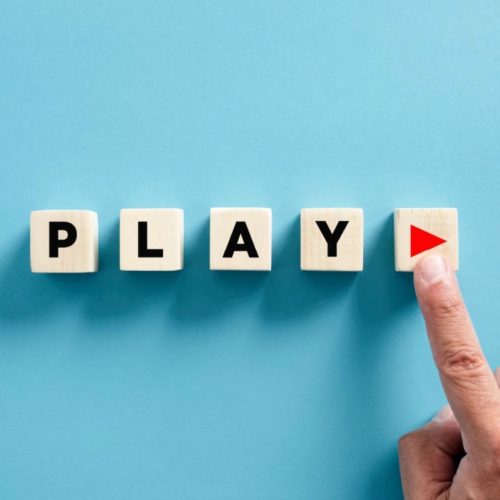
Simplify GPU Usage on Windows with NVIDIA AI Workbench
Discover how NVIDIA AI Workbench eases the use of GPUs for data science, ML, and AI on various systems, offering seamless development, testing, and prototyping opportunities on Windows, macOS, and Ubuntu.
Published 6 months ago on developer.nvidia.com
Abstract
NVIDIA AI Workbench is a user-friendly manager simplifying data science, ML, and AI projects on Windows, macOS, and Ubuntu. It streamlines the setup of GPU-accelerated work through collaboration with ecosystem partners like Canonical and Docker. The tool facilitates managed Docker Desktop installations, offers example projects for quick starts, and supports fine-tuning workflows for models. Future enhancements include app sharing and enhanced multi-container support.
Results
This information belongs to the original author(s), honor their efforts by visiting the following link for the full text.
Discussion
How this relates to indie hacking and solopreneurship.
Relevance
This article is important as it highlights NVIDIA AI Workbench's role in streamlining GPU usage for data science and AI projects on Windows. It showcases opportunities to optimize development environments, leverage Docker for seamless installations, and access example projects for quick setup. Additionally, it introduces future trends like app sharing and multi-container support.
Applicability
If you are working on data science, ML, or AI projects and use GPUs, you should consider utilizing NVIDIA AI Workbench to simplify your development process. Explore the example projects, leverage Docker for easy installations, and stay updated on future enhancements like app sharing and improved multi-container support.
Risks
One potential risk to be aware of is the dependency on NVIDIA AI Workbench for managing GPU-related tasks. If the tool faces technical issues or compatibility challenges in the future, it could disrupt your workflow. Additionally, relying heavily on container technologies like Docker may introduce complexities or dependencies that could impact project stability.
Conclusion
The trend towards simplifying GPU usage for data science and AI projects is likely to continue, with tools like NVIDIA AI Workbench leading the way. Future enhancements such as app sharing and improved multi-container support indicate a user-centric approach to maximizing development efficiency. As an indie hacker, staying informed about these trends can offer insights into optimizing your projects and workflow.
References
Further Informations and Sources related to this analysis. See also my Ethical Aggregation policy.
NVIDIA AI Workbench Simplifies Using GPUs on Windows | NVIDIA Technical Blog
NVIDIA AI Workbench is a free, user-friendly development environment manager that streamlines data science, ML, and AI projects on your system of choice: PC, workstation, datacenter, or cloud.
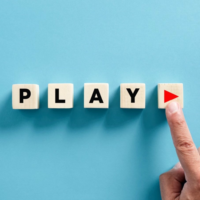
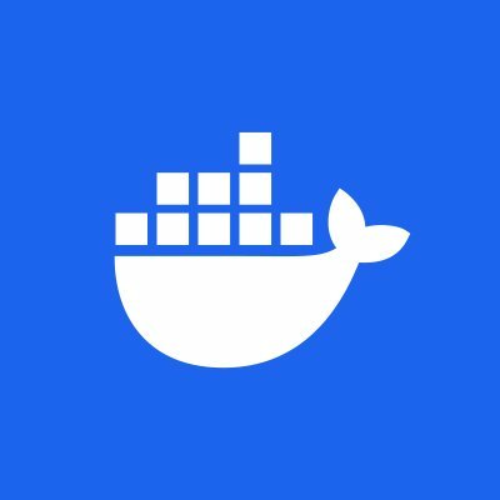
Docker
Stay updated on the latest Docker developments, tips, and best practices to streamline your containerization process. Discover how Docker can enhance your deployment workflow and boost your project's efficiency.
Appendices
Most recent articles and analysises.
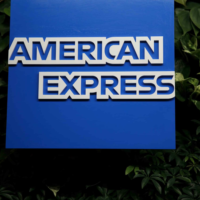
Amex's Strategic Investments Unveiled
2024-09-06Discover American Express's capital deployment strategy focusing on technology, marketing, and M&A opportunities as shared by Anna Marrs at the Scotiabank Financials Summit 2024.