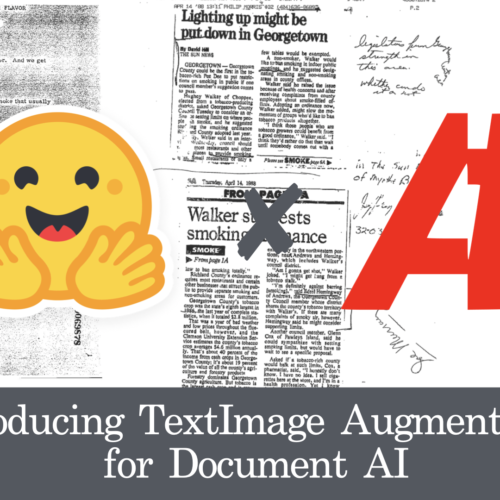
Innovative TextImage Augmentation for Document Images
Learn about the latest data augmentation technique designed to enhance document image processing by integrating text and image modifications. Discover how this approach can revolutionize training models for datasets rich in textual content.
Published 8 months ago on huggingface.co
Abstract
This article introduces TextImage Augmentation, a novel method developed to improve how models interact with document images by combining text and image modifications during training. The technique involves inserting, deleting, and swapping text while altering images, allowing for the creation of diverse training samples. By integrating these augmentations, the pipeline aims to revolutionize document image processing workflows.
Results
This information belongs to the original author(s), honor their efforts by visiting the following link for the full text.
Discussion
How this relates to indie hacking and solopreneurship.
Relevance
This article is crucial for you as it presents a cutting-edge data augmentation technique tailored for document images, offering a solution to enhance text and image interactions in model training. Understanding this method can provide opportunities to improve the accuracy and quality of text extraction in your projects. However, implementing this technique successfully may pose challenges in balancing text and image modifications effectively.
Applicability
To apply the insights from the article, you should consider incorporating the TextImage Augmentation technique into your projects working with document images. By experimenting with text insertion, deletion, swapping, and stopword replacement alongside image transformations, you can generate diverse training samples for improved model training. Make sure to refer to Albumentations AI Documentation for detailed parameters and use cases.
Risks
One potential risk to be aware of is the complexity of balancing text and image modifications effectively. Altering text and images simultaneously may lead to unintended distortions or inaccuracies in the dataset, impacting the model's ability to learn effectively. Additionally, the removal of synonym replacement due to time overhead may limit the diversity of text augmentations.
Conclusion
The long-term trend highlighted in this article is the growing importance of multimodal data augmentation techniques in enhancing AI model performances, particularly in processing document images. Embracing such approaches can lead to more robust models capable of handling datasets with high textual content. As these techniques evolve, they are likely to play a significant role in the future development of AI technologies for document understanding and processing.
References
Further Informations and Sources related to this analysis. See also my Ethical Aggregation policy.
Introducing TextImage Augmentation for Document Images
We’re on a journey to advance and democratize artificial intelligence through open source and open science.
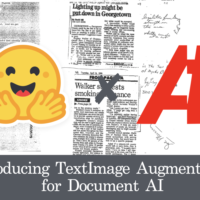
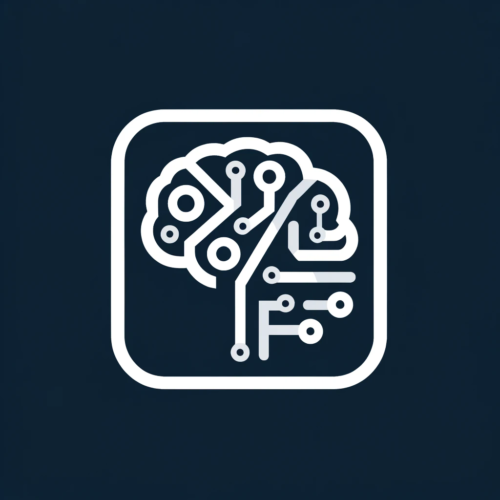
AI
Explore the cutting-edge world of AI and ML with our latest news, tutorials, and expert insights. Stay ahead in the rapidly evolving field of artificial intelligence and machine learning to elevate your projects and innovations.
Appendices
Most recent articles and analysises.
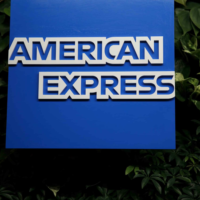
Amex's Strategic Investments Unveiled
2024-09-06Discover American Express's capital deployment strategy focusing on technology, marketing, and M&A opportunities as shared by Anna Marrs at the Scotiabank Financials Summit 2024.